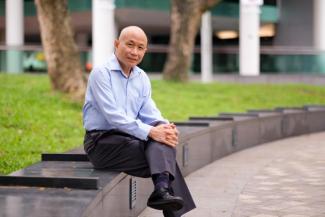
SMU Professor Tse Yiu Kuen is developing econometric models that can provide us with a clearer picture of global markets.
Photo Credit: Cyril Ng
By David Turner
SMU Office of Research– Having broken through most geographical borders, the capital markets have moved on to a new frontier—time. Computing technology has pushed trading well beyond the second-by-second barrier, forcing analysts and investors to grapple with yet another dimension of complexity as they seek to manage their risks.
Mastering the complexities of time, place and market movements is Tse Yiu Kuen, Professor of Economics at the Singapore Management University (SMU). He develops econometric models to isolate the phenomena affecting the tidal flows of the financial world—the inter-continental relationships and daily shifts of stock markets, currency exchanges and interest rates.
Identifying correlated activities across markets
For many people, econometrics conjures up thoughts of esoteric and complicated formulas and algorithms. After all, the application of statistical models to economic theory does seem like an intimidating combination. Yet econometrics could prove essential to our understanding of the markets as the bustle and shouts of trading floors succumb to powerful algorithms trading quietly at a speed and complexity beyond human processing power. A few seconds of activity in New York might significantly affect markets in Singapore or Hong Kong.
High-speed global trading presents a significant challenge for risk management. Even the most basic of investment principles, i.e., not to put all eggs in one basket, is getting more complicated. What if the fate of one basket hangs on the other?
“If two markets are moving in tandem, then you are not diversifying at all,” says Professor Tse. “To know whether they are sufficiently diversifying, financial analysts should understand how different markets are correlated.”
Finding these relationships amongst the tangle of global markets is difficult, but econometrics can shed light on the threads that tie the markets together. Professor Tse has developed econometric models to identify correlated activities among multiple markets, for example between exchanges in Hong Kong and Singapore.
“In the 1990s, some companies moved from Hong Kong’s capital market to Singapore’s,” recounts Professor Tse. “We developed a model to examine changes in Singapore’s Straits Times Index and Hong Kong’s Hang Seng Index before and after this trend, and found a stronger correlation after the companies had moved.”
But even as we get a clearer picture of global markets, they are being wrapped in a fog of speed. Professor Tse says that while no one knows exactly, it has been estimated that high frequency trading forms as much as 70 percent of trading volume in the New York Stock Exchange. Professor Tse’s research helps to clear the fog in delineating the pattern of volatility, a key element of risk.
The lunch break problem
“The great difficulty we have had to overcome is intra-day periodicity. This is a statistical way to describe different trading intensities throughout the day,” he explains with a laugh. “Market activity is very active in the morning, and even though we are looking at New York where they don’t stop for lunch, the activity falls off, then picks up again at closing.”
Analysts need to clear away these daily activity fluctuations to understand the market factors that cause volatility in stock prices, he says. His research has explored various ways of estimating intra-day volatility more reliably, such as in his 2012 paper, “Estimation of High-Frequency Volatility: An Autoregressive Conditional Duration Approach” in the Journal of Business and Economic Statistics. Professor Tse has achieved this by applying a “time-transformation function” method, which transforms real time to hypothetical time.
“This [model] creates a hypothetical day in which activity is uniform from opening to closing, allowing researchers to more accurately estimate volatility,” he describes. “Other methods can only measure volatility over a day, but now we can estimate it over an hour.”
One can use this model to derive a more reliable and accurate assessment of whether other factors, such as insider trading and regulatory requirements, are causing volatility, says Professor Tse.
The ‘volatility smile’
Using this model, Professor Tse’s empirical analysis has found that intra-day volatility exhibits a ‘volatility smile’, and his methods allow us to see the real smile rather than one distorted by daily activity fluctuations, as explained in his 2014 paper, “Intraday periodicity adjustments of transaction duration and their effects on high-frequency volatility estimation” published in the Journal of Empirical Finance.
Volatility is highest in the morning. It then drops in the afternoon but rises to complete the smile at the end of the day. “Traders are reacting to news that have accumulated over the night; and then towards closing, they are digesting what has happened over the course of the day,” he says.
According to Professor Tse, intra-day volatility affects high frequency traders who go in and out of the market many times a day, instead of traditional traders, such as those who operate mutual funds and generally trade far less frequently.
In fact, Professor Tse’s model is most useful to traders who need to estimate their ‘Value-at-Risk’ over a short intraday period, which is also known as ‘intraday VaR’. While traditional financial analysts assess the volatility risks in their portfolio day-by-day or week-by-week, high frequency traders may need to know their volatility exposure over a horizon as short as three hours.
Even within this narrow window, real time analysis is possible, he says. “Although it requires a lot of steps, my model can be used in real time, using the Monte Carlo method,” he explains, referring to the statistical technique of generating hypothetical data many times over to estimate what might happen in reality. And Professor Tse’s work is improving the risk management tools available to analysts in this short timeframe. He demonstrates how his method outperforms earlier models in a forthcoming paper, “Intraday Value-at-Risk: An asymmetric autoregressive conditional duration approach” to be published in the Journal of Econometrics.
Fortunately, data is not a problem. For a decade now, stock exchanges have opened up trading data in real time, so researchers can study ‘tick by tick’ data to examine trends, be it over a year or a day.
But even econometric models require more developments in order to catch up with the speed of modern trading, says Professor Tse, with an eye to the future of his field. “Our models deal with second-by-second trading, but now high frequency trading happens nano-second by nano-second. That is the future for our research.”