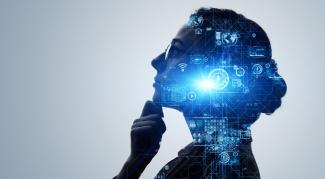
Artificial Intelligence (AI) based systems are increasingly being embedded into more aspects of our everyday lives as consumers and as employees. Worldwide, there are fears of whether further advancement and increased diffusion of AI capabilities will take over our livelihoods, and even concerns of what this all means in terms of being human.
In a webinar held on 25 August, Professor Emeritus Steven Miller talked about how AI can and should be embraced. Organised by Singapore Management University’s Business Partnerships unit and International Trading Institute, the thought leadership session brought together industry partners, faculty and students to delve into the issue of ‘Working with AI-Enabled Smart Machines’.
Over an 18-month period (January 2020 through June 2021), Professor Emeritus Miller worked with noted U.S author and Business-IT thought leader Professor Tom Davenport to document 30 examples of people doing their everyday work in real-world business settings in partnership with AI-enabled smart machines. These case studies will be used in their co-authored book ‘The Future of Work Now: People Collaborating with Smart Machines’, to be published by MIT Press in 2022.
The case studies covered a range of industry settings including insurance and financial services, knowledge work across other service sector industries, healthcare, factory floor production, and field operations across multiple industries. During the webinar, Professor Miller shared several examples and insights from case studies of Singapore-based companies.
One example cited was from Development Bank of Singapore (DBS)’s massive migration to data analytics starting in 2010 and their follow-on progression into using machine learning. He spoke about a DBS system which is able to draw on the bank’s existing data sources and external data to evaluate the probability of fraud or financial crime. Prior to using this new system, the majority of the time spent by the bank employees who were doing transaction surveillance was on data amalgamation and sorting through the alerts generated by the prior generation of rule-based systems. The latter is an earlier type of AI application, with most of these alerts being false alarms (false positives).
With the new machine learning based system analysing and evaluating the rule-based alerts, the transaction surveillance employees can now focus directly on the alerts identified by the system as having a high or medium probability of being an actual problem. The employee’s work time is allocated more efficiently, as they no longer need to look at large numbers of false alarms. Additionally, they no longer need to manually amalgamate all the supporting information use to evaluate each alarm as that background data access and integration work was automated as part of the machine learning application.
An important takeaway from this case study was that even though the productivity of the employees doing transaction surveillance substantially increased, the number of employees doing this type of work was not reduced. Rather, DBS management used this recouped time and capacity to enable their transaction surveillance employees to spend more effort on higher value-add work. They are now able to spend more time investigating transactions which are actually suspicious, on keeping abreast of new types of financial fraud and crime trends, and on working with the technology team to better detect new types of financial crimes.
Professor Emeritus Miller also highlighted a case study he did on Shopee, Southeast Asia’s largest e-commerce platform. They are a digital-native born company whose business is based on AI-enabled data analytics. The Shopee case study highlighted the role of their product managers. These are the people who orchestrate the complex process of developing, phasing in and scaling new Shopee e-commerce platform capabilities and feature enhancements. The product manager’s challenge is to do this in a way that meets business goals, satisfies customer needs, deals with the constraints and problems faced by the technology teams developing the new AI-enabled capabilities and features, and addresses the many conflicting requirements and trade-offs that arise.
The case study highlighted that while product managers are overseeing the processes of bringing AI-enabled capabilities and features of the Shopee platform to market, the nature of their role is so multi-faceted and complex that very few of their engagement management, negotiation, coordination, and decision-making tasks can be automated by these same type of AI capabilities. This product manager example illustrates one of the important ways in which human roles are required to manage the implementation of AI-based change efforts within a complex company setting.
The typical Shopee product manager came from a more business-oriented background and worked for the product management unit that was part of the “business-side” of the organisation. The company also had a few product managers who were computer science trained, previously worked on building AI-based prediction models, and migrated into the product manager role though based within the Data Science team. They would team with the more business-oriented product manager. Because they had in-depth knowledge of the capabilities of the AI-models, and more direct access to the company’s data as a result of being part of the Data Science team, these more technically oriented product managers were able to see possibilities for how AI could be used to create new capabilities and features that people on the business side were not aware of or would not have asked for.
As a result, these product managers within the Data Science team were able to make “bottom up” suggestions for new product capabilities and features that never would have arisen through the more typical “top down” process of having the business product manager give the requirements to the Data Science team. The case highlighted that companies that are able to have both top-down and bottom-up sources of suggestions for new AI-based product capabilities and features would be in better shape for improving sales and staying competitive.
The third local company highlighted was Certis Group, which provides security and service support to many large buildings and properties such as airports, shopping malls, commercial buildings, and industry parks. The case study setting was Changi Jewel Airport where Certis provides support for physical security, guest services and facilities maintenance. With the deployment of AI, the use of thousands of sensors, a centralised Smart Operations Centre to serve as mission control for monitoring, communication and coordination, and an intelligent mobile app to support all ground staff, Certis is able provide support services over the entire Jewel Mega-mall facility more comprehensively and in an economically viable way. Without the use of these supporting technologies, it would not have been possible to provide the necessary support services in a cost effective way as it would have required far more ground staff and even then, it would not have been possible to monitor and manage this very large multi-use facility.
Through digitalisation and AI enablement, the company created a mobile app tool to support all of the ground staff. This mobile app substantially simplified the process of creating and submitting incident reports. Submission of incident reports had always been a time consuming and challenging task for the security officers and other ground support staff, especially so for older employees and those who were not able to adequately write reports in English. Because of the simplified ability to submit an incident report, and all of the other support information provided by the mobile app, the security officers, the guest service officers, and the facility maintenance workers are able to provide cross-functional support for one another. This provides expanded job scope and variety, and leads to better ground support. This was a good example of how digitalisation and AI-enabled technology added to the job content of the front-line worker, and also how the technology can provide support for older workers in ways that enable them to remain in their jobs.
Summing up the webinar, Professor Emeritus Miller reiterated that in this ongoing wave of using data-driven, machine-learning-based AI in the workplace, human work is not going away: not now, not in the next several decades to come, and likely not even in the longer term. While some jobs—a relatively small fraction-- will be entirely automated and eliminated, most jobs will end up being changed and transformed, but will still exist and be made more productive with AI. He also highlighted that while improvements in AI methods and technology are developing fast, deployment of AI within organisations is always a complex and slow process.
He concluded by noting that the threat is not about AI taking away our jobs. “The real threat is when we choose not to team with AI.” He also emphasised that organisations need to learn how to capitalise on what AI can do, go beyond just thinking about simple labour displacement and manpower cost savings, and find ways to use the technology to create value in ways that leads to new demand and correspondingly to new employment opportunities. And while companies do this, they need to ensure that they share the benefits of increased productivity and value creation with their employees who are the ones using the new technology on behalf of the company to achieve these gains.”