Leveraging AI to boost resource management
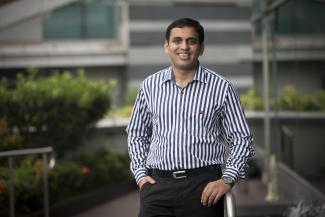
Artificial intelligence and machine learning technology are being applied in an increasingly broad range of applications in the areas of transportation and security. In a series of papers, Associate Professor Pradeep Varakantham from the Singapore Management University and fellow researchers have proposed AI-based solutions that aim to improve the effectiveness of screening passengers at ports of entry, as well as optimise on-demand ride pooling and matching services for transportation service providers.
Screening threats more effectively
The ability to conduct large-scale screening for potential threats is a problem at many airports, seaports, and other ports of entry. Adversaries can observe screening procedures and choose to arrive when there are gaps in screening due to limited resources. Ports use a model known as a Threat Screening Game (TSG) to attempt to address this issue. “There is not enough time to screen every passenger and their luggage. Given the limited time, TSG studies the problem of what screening resources can be used to screen people on different flights so as to minimise the risk of missing a threat,” said Assoc Prof Varakantham.
Existing methods recommend using the same screening strategy for all passengers arriving within an hour. In practice, however, airport passengers arrive in bursts and are not bound by a fixed window of time.
To address this, Assoc Prof Varakantham, with fellow team members from SMU’s School of Information Systems (SMU SIS) and Harvard University’s Center for Research on Computation and Society, propose a screening strategy which is based on earlier passengers who have passed through screening. This means that all high-threat passengers on a certain flight have already been screened before the flight takes off, leaving only the low-threat passengers to be screened upon arrival at the destination airport.
“Due to this new method, we reduce the waiting time for passengers without increasing the risk of missing a threat. Also, unlike previous methods, our method can be used in real-time,” he said.
On-demand ride-pooling
On-demand ride-pooling services such as GrabShare have become increasingly popular because of its ability to lower costs for passengers while also increasing revenue for drivers. To ensure near real-time response, existing ride-pooling solutions aim to maximise the number of passengers served without considering how a current assignment can impact future assignments.
“Given the tight time constraints, existing methods consider assigning the nearest passenger to the nearest cab, without considering whether that assignment will result in getting more customers in the same taxi,” said Assoc Prof Varakantham.
“For instance, a customer who wishes to go to Tuas from the city centre is likely to be assigned the vehicle that is closest, but assigning this particular vehicle may not consider the fact that there are likely to be few passengers interested in going to Tuas.”
However, considering the future effects of an assignment adds an extra layer of complexity when attempting to optimise ride-pooling. Assoc Prof Varakantham and his team from SMU SIS propose using a novel combination of machine learning methods to compute and match current demand to current supply, while planning for potential future requests that can be picked up along the way for pooling. “We show that our approach easily outperforms leading approaches for on-demand ride-pooling on a real-world dataset by up to 16 per cent. This can improve the revenue earned by aggregation companies and individual drivers as well,” he said.
Matching transport services online
A similar issue exists in the online matching of services to customers in shared transportation and delivery services. In this case, the matching of services to customers in one round has a direct impact on the matching of services to customers in the next round. For instance, taxis in the second round of matching can only pick up customers closer to the drop-off point of the customer from the first round of matching.
“Similar to ride-pooling, existing matching methods only match current supply to current set of requests.
These methods are able to achieve about 60 per cent of the best outcomes. Although this is good, it can be improved significantly,” said Assoc Prof Varakantham.
As such, a solution proposed by a team made up of members from SMU SIS and Massachusetts Institute of Technology’s Department of Electrical Engineering and Computer Science, considers potential future requests while matching the supply and demand of shared transportation and delivery services at the current time step.
“Our approach was pilot-tested with a group of taxi drivers in finding customers, based on live streaming data of all taxi drivers in Singapore. We obtained an improvement of 28 per cent over drivers who did not have any guidance.”