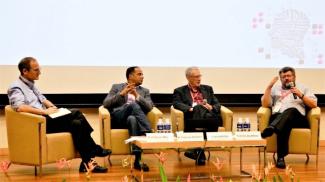
Beyond the hype surrounding each technology, artificial intelligence and blockchain could have a synergistic impact when used together.
Above (from left): SMU Vice Provost (Research) Professor Steven Miller; Mr Sopnendu Mohanty, Chief FinTech Officer of the Monetary Authority of Singapore; Professor John Hopcroft, IBM Professor of Engineering & Applied Mathematics in Computer Science at Cornell University; Professor Efim Zelmanov, Professor of Mathematics at the University of California, San Diego.
By Rebecca Tan
SMU Office of Research & Tech Transfer – Fuelled by the dizzying rise of cryptocurrency prices and pithy pronouncements such as “AI is the new electricity”, artificial intelligence (AI) and blockchain have become two of hottest topics of discussions in newspapers and around office water coolers. Although both AI and blockchain are probably at the peak of the ‘hype cycle’ at the moment, that is where their similarities end; these two technologies actually represent contrasting ways of understanding and ordering the world.
“In a way, blockchain is about certainty and transparency; a permanent and publicly verifiable way to record transactions,” said Professor Steven Miller, Vice Provost of Research at Singapore Management University (SMU). “AI, on the other hand, involves making probabilistic statements and in that sense can be said to be about uncertainty.”
Professor Miller made these comments as moderator of a panel discussion titled ‘When Blockchain & AI Come Together: Possibilities & Impacts,’ held on 24 January 2018 at SMU. Organised by SMU in conjunction with the National Research Foundation’s Global Young Scientists Summit, the panel comprised three distinguished speakers: Mr Sopnendu Mohanty, Chief FinTech Officer of the Monetary Authority of Singapore; Professor John Hopcroft, IBM Professor of Engineering & Applied Mathematics in Computer Science at Cornell University (Turing Award recipient, 1986); and Professor Efim Zelmanov, Professor of Mathematics at the University of California, San Diego (Fields Medallist, 1994).
Never the twain shall meet?
“In my understanding, AI looks for patterns in a large amount of data, while blockchain shows you what data is there so that you don’t have to second guess what is happening; you just trust your data,” Mr Mohanty said.
If implemented properly, distributed ledger technologies (DLT) such as blockchain would actually reduce financial institutions' need for AI, he added. “Central banks and other regulators are interested in using AI to monitor the financial system, to catch bad behaviour and market manipulators. But if all market transactions are conducted on a DLT platform, there might not be as large a need to use AI for that purpose.”
AI and blockchain are thus two different ways of achieving the same outcome – in this case, a sound and secure financial system, said Mr Mohanty.
Agreeing, Professor Hopcroft added that not all problems need to be solved with AI. “Deep learning works well for a particular class of problems – those that involve pattern recognition in multi-dimensional space. If you have a problem of that nature, then deep learning is certainly the way to solve it.”
“But deep learning is not helpful if your problem does not involve pattern recognition,” said Professor Hopcroft, who won the Turing Award in 1986 for his work on the design and analysis of algorithms and data structures. “Other classes of problems, including things such as verifying property ownership, can be solved by having the transactions recorded on the blockchain.”
Convergence on the horizon
Given these fundamental differences between AI and blockchain, you might not think that there is any way to combine them fruitfully. On the contrary, the possibilities are nearly endless, the panel members said, and are limited only by our ability to imagine ways to use the strengths of each technology to complement the drawbacks of the other.
One potential point of convergence raised was the possibility of using the transparency of blockchain to unlock more data for AI. AI is fuelled by having access to large amounts of data. However, countries are becoming increasingly nationalistic about digital data generated by their citizens and may soon clamp down on data transmission across borders, Mr Mohanty said.
“Very soon, big data could become small data, leaving AI researchers only domestic data to play with. For policy makers, there is a mismatch between protecting their citizens and capturing the opportunities that AI offers,” he said.
One way around this impasse is for data to be shared through the blockchain. Through the use of protocols such as zero-knowledge proofs, it is possible to selectively share information on the blockchain without revealing the identity of the person to whom the data belongs, said Professor Hopcroft.
“In healthcare, for example, blockchains could help us store information in such a way that doctors can see a patient’s entire medical history, but researchers are only shown statistical data instead of any personal information,” he said. This possibility is still theoretical, he cautioned, as cryptographic researchers are still working on a way to scale up zero-knowledge proofs in an efficient manner.
On the other hand, blockchains could also prevent data from being monopolised, said Professor Zelmanov. “The concentration of data gives power. Distributed ledgers can help prevent the concentration of data in the hands of a few,” he said.
Realising technology’s promise
Although it has been ten years since the original Bitcoin white paper was released, the cryptocurrency has still not achieved its stated goal of being an efficient payment system, Mr Mohanty said. Blockchain technologies, therefore, have yet to deliver on their promise of being efficient decentralised systems, he added.
Despite all the ways in which AI has already been successful in solving problems in a wide range of disciplines, a subset of AI methods for machine learning – neural networks with multiple layers (deep learning) – has been criticised for being a ‘black box’ in that it is not completely clear how algorithms arrive at a decision.
“But it shouldn’t bother you too much that we don’t know why this works – this is the nature of engineering,” Professor Hopcroft said. “When we first made airplanes fly, we didn’t really understand aerodynamics, but we subsequently developed the theory and used it to improve airplanes.” The implication of Professor Hopcroft’s comment is that as we continue to use neural networks, scientists will figure out how these ‘black box’ methods arrive at their decisions.
“With AI and blockchain, we’ve discovered technologies that are very important and will be used extensively in industry. As we go along, I think we will figure out why they work and make them work much better,” Professor Hopcroft concluded.
Back to Research@SMU Issue 52
Image credit: Rebecca Tan