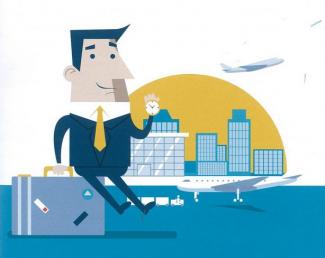
![]() |
MA Nang Laik Assistant Professor of Information Systems (Practice) Director, MITB (Analytics) Programme |
Growth in air traffic has been remarkable despite economic recession cycles. Air passenger traffic increased an average of about 6% worldwide in the third quarter of the twentieth century, which means the volume of air travel has doubled every 10 to 15 years. Airport terminals face a capacity issue as the number of air travellers increases.
Most airlines do not submit information about passenger load to airports in advance because sale of airline tickets continues till the day of departure. Most of this information is only available to airport operators a few hours before a flight departs or arrives. From the perspective of airport operators though, the number of passengers arriving at and departing from an airport is one of the most crucial factors required for planning and resource allocation. Without concrete figures, airport operators can only assign the number of check-in counters required daily based on "gut-feel" and experience. Overestimation of passenger load means that unnecessary resources, such as check-in counters with low utilisation will be wasted, and this wastage translates into higher operational costs. On the other hand, underestimation will result in long passenger queues at check-in counters and failure to meet service level agreements with the various airlines.
A major Asian airport operator is facing challenges of increasing passenger traffic, limited terminal resources to deal with check-ins, and long waiting time at check-in counters that result in falling passenger satisfaction levels. To solve these problems, the operator wants to use analytics to glean useful insights from past trends and travelling patterns to predict passenger loads and improve operational efficiency. For example, a forecast of passenger load can help determine the number of check-in counters that need to be opened during a particular period.
Our analytics team observed that passenger load varies according to day of the week, destination city and seasonality. We then built a passenger load forecast model using a decision tree with the following variables as input: day of week, destination city and month of the year. The model is then validated over one month using "live" data by observing the difference between actual passenger load and our predicted load. We have achieved a very high accuracy rate with root means squares error of 10% for all airlines.
However, our prediction model has two limitations: it assumes that past demand is a good estimation of current passenger load. The model also does not take into consideration external factors such as airline promotions, "long weekend" holidays, new destinations and airline growth.
The predicted passenger load from the model was also used as input in another simulation model that mimics the arrival pattern of airline passengers for departure process. The simulator can be used to predict the average waiting and serving time at each check-in counter — a very important factor to consider when meeting service level agreements. Airport operators can perform "what-if" analysis to identify the optimal number of check-in counters required to balance resources and queuing time, without breaching quality standards.
The model developed has been used by terminal managers in daily operations at the airport to better estimate each airline's passenger load, which is then used to better manage resources such as check-in counters more efficiently. The forecast passenger load was also used in other business decisions by various airlines using the airport. Through this project, predictive and prescriptive analytics has been applied very successfully in assisting the airport operator to utilise its terminal resources more effectively, thus reducing operational costs and improving passenger check-in experiences at the airport.
Understanding Different Analytics
Descriptive Analytics provides information on or a summary of a sample of data, using basic statistical measures. Some examples of descriptive analytics are finding mean, median, variance, standard deviation or histograms. It helps us to answer questions such as, what has happened, how, and why did it happen? It helps us to understand the current stage of art and business process, but doesn't tell us what will happen in future or be reactive.
Predictive Analytics involves advanced modelling, machine learning and data mining techniques to identify patterns in the data so that the decision makers can predict what is going to happen in the future. The models can find the relationships between the input factors, which are then used to predict the output (dependent) variables such as credit score in the financial industry, using customer demographic, incomes and spending.
Prescriptive Analytics is the final stage of analytics and specifies courses of action to achieve the predictive outcome. It involves using complex algorithms such as optimisation or simulation techniques to minimise risk or optimise resource utilisation or achieve the best outcome based on predictions or recommendations.
This article is reproduced from The IT Society, the official publication of the Singapore Computer Society, under CC license 3.0