Understanding human languages using computational methods
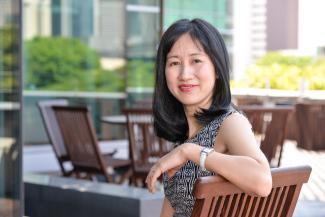
Prof Jiang Jing from the School of Computing and Information Systems is a respected researcher and academic in natural language processing (NLP), a key subfield of Artificial Intelligence that aims to understand human languages using computational methods. She has investigated broadly on the applied side of NLP, proposing new solutions based on principled machine learning models to problems in a range of areas including information extraction, topic modelling, sentiment analysis, social media analysis, and most recently question answering.
Prof Jiang said, “A central concern that motivated my selection of research problems is that I see a prevalent and pressing need in real-world applications for advanced language technologies to quickly discover trends and patterns and to accurately extract knowledge from the huge amount of textual data surrounding us today. To address this pressing need, I have developed novel solutions to push the state of the art of language technologies.”
A current topic she is researching on is the study of AI models especially for visual and verbal question-answer systems. This is necessary to enable machines to work together with people interactively through natural communications for joint problem solving.
Prof Jiang has published over 100 papers, many in top-tier conferences and journals. This is evidenced by the more than 14,500 citations listed on Google Scholar, corresponding to an H-index of 45, which would be termed as “outstanding”.
One example is “Improving Multi-hop Knowledge Base Question Answering by Learning Intermediate Supervision Signals”. Published in 2021, the paper has received twenty times more citations than other similar papers.
This paper deals with the problem of answering questions using knowledge stored in so-called "knowledge graphs", which store entities such as people and organisations together with their relations such as “X is the CEO of company Y and company Y was acquired by company Z”. Although answering straightforward questions such as "who is the CEO of Google" is relatively easy, when a question is longer and involves multiple relations, such as "who is the founder of the company that was first acquired by Google," the task becomes much more complex, requiring more computation and producing less accurate results because of the propagated errors through multiple steps of reasoning. From the machine learning standpoint, the major technical challenge here is the lack of supervision signals at intermediate steps.
To address this challenge, Prof Jiang and her team adopt the curriculum learning framework and propose a novel teacher-student approach for the multi-hop knowledge base question answering task. In their approach, the student network aims to find the correct answer to the question, while the teacher network tries to learn intermediate supervision signals for improving the reasoning capacity of the student network. The main novelty lies in the design of the teacher network, where we utilise both forward and backward reasoning to enhance the learning of intermediate entity distributions. By considering bidirectional reasoning, the teacher network can produce more reliable intermediate supervision signals, which can alleviate the issue of spurious reasoning. Extensive experiments on three benchmark datasets have demonstrated the effectiveness of our approach on the Knowledge Base Question Answering task.
She has also chaired and spoken at various academic conferences, such as being a keynote speaker at the International Conference on Computational Linguistics and Intelligent Text Processing in 2018.
In a global study by Stanford University in 2020, Prof Jiang was recognised as among the top 2% of scientists in the world in the field of Artificial Intelligence & Image Processing.
Her other accolades include:
- 2021 Singapore 100 Women in Tech List
- ECIR 2021 Test of Time Award at the 2021 European Conference on Information Retrieval (ECIR) for her co-authored paper titled “Comparing Twitter and Traditional Media Using Topic Models,” published at ECIR 2011
- WSDM 2020 Test of Time Award at the 2020 ACM International Conference on Web Search and Data Mining (WSDM) for her co-authored paper titled “TwitterRank: Finding topic-sensitive influential Twitters,” published at WSDM 2010
- Lee Kuan Yew Fellowship for Research Excellence, Singapore Management University, 2020
- Lee Kong Chian Fellowship, Singapore Management University, 2017
Besides her teaching responsibilities, Prof Jiang was Deputy Director of the Living Analytics Research Centre (LARC) conducting research on large-scale data analytics to support Singapore's Smart Nation initiatives. She is currently Director of the Artificial Intelligence & Data Science Cluster of the School of Computing and Information Systems.
At LARC, Prof Jiang and her team focused on social sensing and question answering. Some of the research projects include:
(1) Developing several question answering algorithms which achieved state-of-the-art performance on benchmark datasets. Papers documenting these algorithms have also received many citations.
(2) Collaborating with the Institute of Policy Studies (IPS) to study sentiments observed in Singapore’s online social media space. Studies done included the analysis of online sentiments after Prime Minister’s National Day Rally Speech and analysis of Singapore online users’ social media consumption patterns to help IPS study online falsehoods.
(3) Working with the Municipal Services Office (MSO) to study the feasibility of using online social media to gather citizen feedback in real time.
(4) Working with Professor Paulin Straughan on an AISG 100 Experiment project that tries to use AI to predict dating preferences. This is in collaboration with a Singapore-based company providing dating services in the region.
“Looking ahead, I plan to continue my research in a few directions that I believe will push the frontiers of NLP. A promising direction to enhance AI's capabilities to communicate and collaborate with humans is multimodal interactions, combining vision, speech, text and other modalities of communication channels to enable human-like interactions between machines and humans. Our understanding of fusing different modalities of signals for reasoning and prediction such as answering questions expressed in language based on information contained in images is still highly limited, despite the progress made in recent years. Another fundamentally important direction is to develop explainable NLP and AI models, which is critical for identifying limitations with existing deep learning models and injecting higher-level intelligence into AI,” Prof Jiang added.